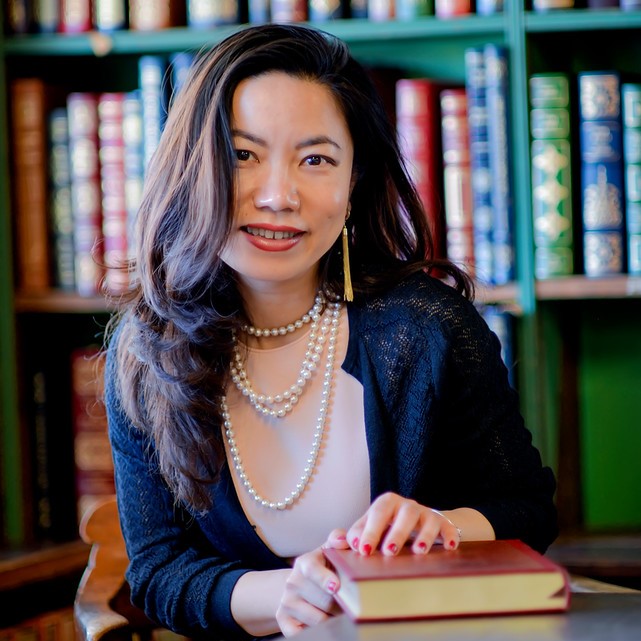
Dr. Yi Huang is an NIH Data and Technology Advancement (DATA) National Service Scholar. She also serves as an associate professor and the former graduate program director of statistics in the Department of Mathematics and Statistics at the University of Maryland Baltimore County. She received her Ph.D. in biostatistics from the Johns Hopkins Bloomberg School of Public Health in 2007, specializing in biostatistical methodology and causal inference research.
Dr. Huang’s project at NIBIB focuses on enhancing the quality of our nation’s over-the-counter (OTC) COVID-19 home testing dataset. Dr. Huang will conduct comprehensive analyses to understand human behaviors related to home self-test reporting, address health disparity questions surrounding self-testing, and explore the opportunities and limitations of using home self-test data for nationwide disease prediction models and regulatory decision-making. Dr. Huang’s project aims to fill the gap in understanding the possibilities and limitations for leveraging self-reported OTC testing data with traditional laboratory and hospital data in building a comprehensive public health surveillance system. As future OTC tests emerge for additional diseases (e.g. influenza, STDs), her project will play an important role in improving future health monitoring and disease prevention.
As a biostatistician, Dr. Huang has a diverse set of experiences in research, ranging from designing analytical plans and conducting statistical analyses for medical applications to developing new statistical methods and models that address new design features or new data formats. In particular, her current research interests include causal inference methodology on treatment effect estimation dealing with covariate measurement error, synthetic control arm construction using external data, advanced meta-analysis for rare events, causal meta-analysis addressing study heterogeneity across randomized controlled trials, treatment effect efficacy and safety assessment using real world evidence, and the biostatistics research projects in gerontology, cardiovascular disease, and cancer diagnosis.