- What is computational modeling?
- How is computational modeling used to study complex systems?
- How can computational modeling improve medical care and research?
- How are NIBIB-funded researchers using computational modeling to improve health?
What is computational modeling?
Computational modeling is the use of computers to simulate and study complex systems using mathematics, physics and computer science. A computational model contains numerous variables that characterize the system being studied. Simulation is done by adjusting the variables alone or in combination and observing the outcomes. Computer modeling allows scientists to conduct thousands of simulated experiments by computer. The thousands of computer experiments identify the handful of laboratory experiments that are most likely to solve the problem being studied.
Today’s computational models can study a biological system at multiple levels. Models of how disease develops include molecular processes, cell to cell interactions, and how those changes affect tissues and organs. Studying systems at multiple levels is known as multiscale modeling (MSM).
How is computational modeling used to study complex systems?
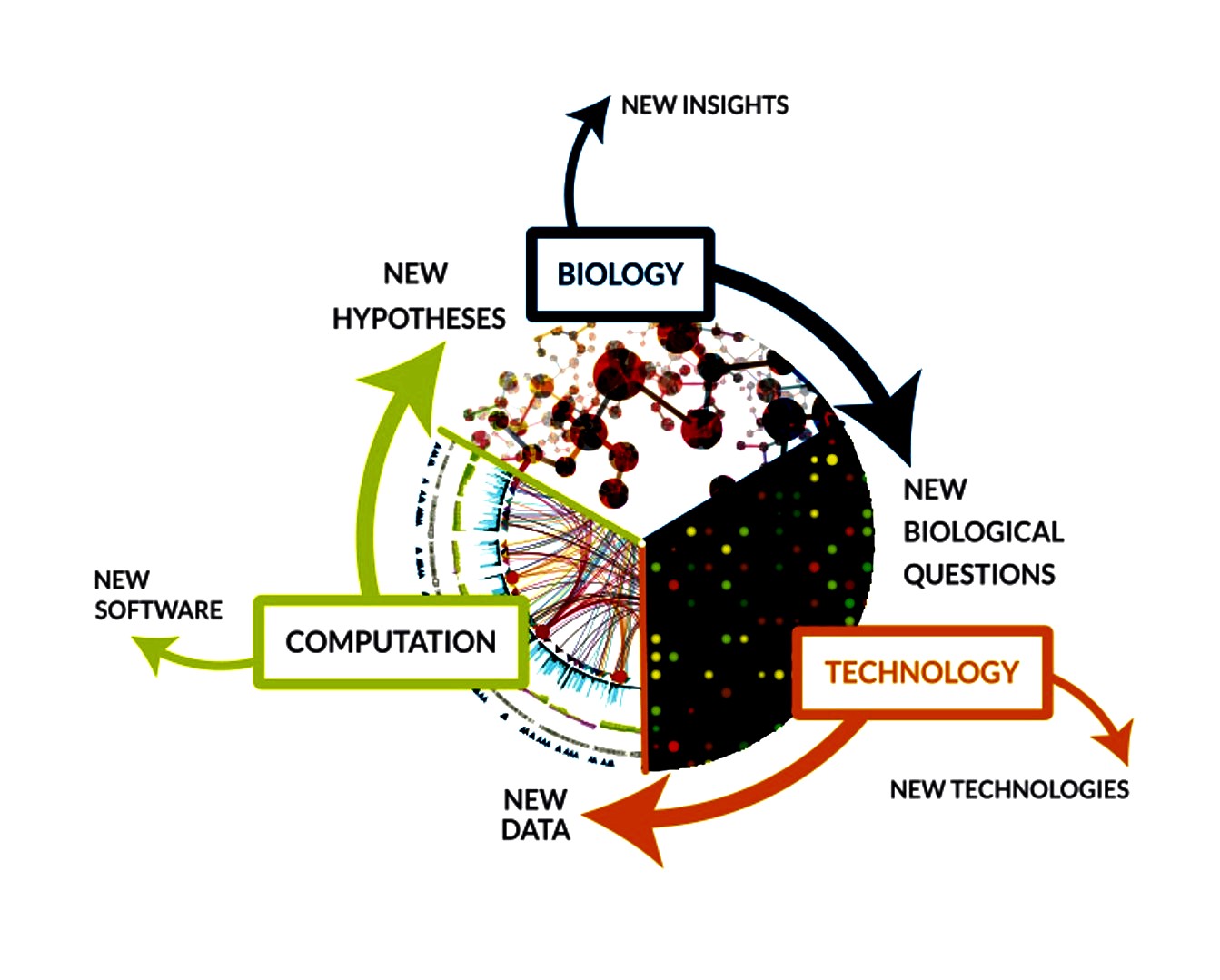
Weather forecasting models make predictions based on numerous atmospheric factors. Accurate weather predictions can protect life and property and help utility companies plan for power increases that occur with extreme climate shifts.
Flight simulators use complex equations that govern how aircraft fly and react to factors such as turbulence, air density, and precipitation. Simulators are used to train pilots, design aircraft, and study how aircraft are affected as conditions change.
Earthquake simulations aim to save lives, buildings, and infrastructure. Computational models predict how the composition, and motion of structures interact with the underlying surfaces to affect what happens during an earthquake.
How can computational modeling improve medical care and research?
Tracking infectious diseases. Computational models are being used to track infectious diseases in populations, identify the most effective interventions, and monitor and adjust interventions to reduce the spread of disease. Identifying and implementing interventions that curb the spread of disease are critical for saving lives and reducing stress on the healthcare system during infectious disease pandemics.
Clinical decision support. Computational models intelligently gather, filter, analyze and present health information to provide guidance to doctors for disease treatment based on detailed characteristics of each patient. The systems help to provide informed and consistent care of a patient as they transfer to appropriate hospital facilities and departments and receive various tests during their course of treatment.
Predicting drug side effects. Researchers use computational modeling to help design drugs that will be the safest for patients and least likely to have side effects. The approach can reduce the many years needed to develop a safe and effective medication.
How are NIBIB-funded researchers using computational modeling to improve health?
Modeling infectious disease spread to identify effective interventions. Modeling infectious diseases accurately relies on numerous large sets of data. For example, evaluation of the efficacy of social distancing on the spread of flu-like illness must include information on friendships and interactions of individuals, as well as standard biometric and demographic data. NIBIB-funded researchers are developing new computational tools that can incorporate newly available data sets into models designed to identify the best courses of action and the most effective interventions during pandemic spread of infectious disease and other public health emergencies.
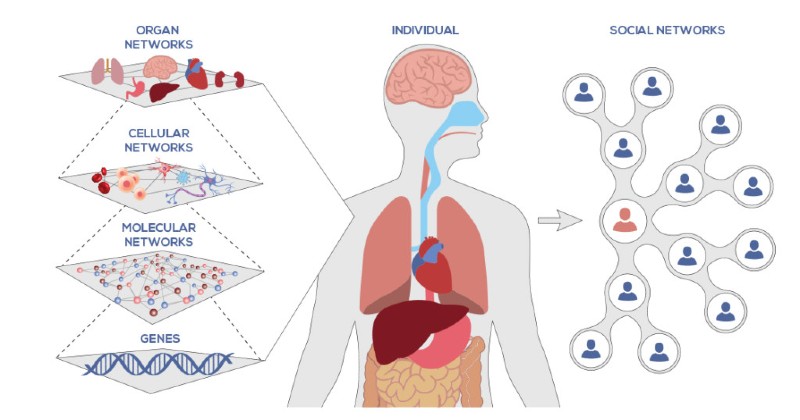
Tracking viral evolution during spread of infectious disease. RNA viruses such as HIV, hepatitis B, and coronavirus continually mutate to develop drug resistance, escape immune response, and establish new infections. Samples of sequenced pathogens from thousands of infected individuals can be used to identify millions of evolving viral variants. NIBIB-funded researchers are creating computational tools to incorporate this important data into infectious disease analysis by health care professionals. The new tools will be created in partnership with the CDC and made available online to researchers and health care workers. The project will enhance worldwide disease surveillance and treatment and enable development of more effective disease eradication strategies.
Transforming wireless health data into improved health and healthcare. Health monitoring devices at hospitals, and wearable sensors such as smartwatches generate vast amounts of health data in real-time. Data-driven medical care promises to be fast, accurate, and less expensive, but the continual data streams currently overwhelm the ability to use the information. NIBIB-funded researchers are developing computational models that convert streaming health data into a useful form. The new models will provide real-time physiological monitoring for clinical decision making at the Nationwide Children’s Hospital. A team of mathematicians, biomedical informaticians, and hospital staff will generate publicly shared data and software. The project will leverage the $11 billion wireless health market to significantly improve healthcare.
Human and machine learning for customized control of assistive robots. The more severe a person’s motor impairment, the more challenging it is to operate assistive machines such as powered wheelchairs and robotic arms. Available controls such as sip-and-puff devices are not adequate for persons with severe paralysis. NIBIB-funded researchers are engineering a system to enable people with tetraplegia to control a robotic arm while promoting exercise and maintenance of residual motor skills. The technology uses body-machine interfaces that respond to minimal movement in limbs, head, tongue, shoulders, and eyes. Initially, when the user moves, machine learning augments the signal to perform a task with a robotic arm. Help is scaled back as the machine transfers control to the progressively skilled user. The approach aims to empower people with severe paralysis and provide an interface to safely learn to control robotic assistants.
Updated May 2020