- What are the types of AI?
- How is AI being used to improve medical care and biomedical research?
- How are AI datasets created?
- How are NIBIB-funded researchers leading the way in the development and application of AI in biomedicine?
What are the types of AI?
Artificial Intelligence: The ability of machines to learn through experience and gain proficiency through examples, not just by carrying out computations as instructed by human users.
- Early applications of AI included machines that could play games, such as checkers and chess, and programs that could reproduce language.
Machine Learning: An approach to AI by which a developer devises a computer algorithm — a set of rules and procedures — to analyze data and make predictions. Machine learning tools identify hidden patterns within large collections of data and then can make predictions.
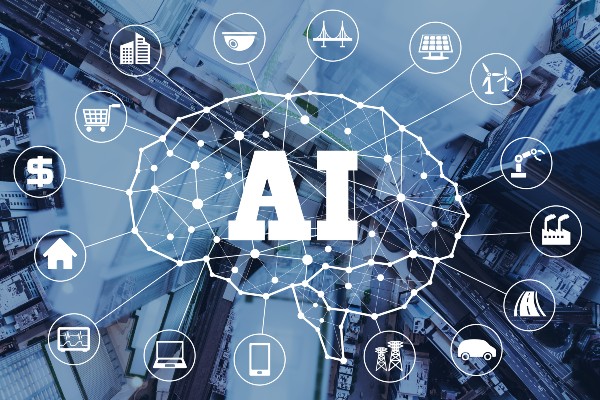
- Machine learning-based technologies are used every day in tools from personalized news feeds to financial fraud detection.
Neural Networks: A machine learning approach modeled on the human brain in which algorithms process signals via interconnected layers of artificial neurons.
- Mimicking biological nervous systems, artificial neural networks have been used to recognize and predict patterns of neural signals involved in brain function, especially in differentiating typographic characters and in recognizing facial features.
Deep Learning: A form of machine learning that uses many layers of computation to form a more complex neural network — often called a deep neural network — that is capable of learning from large amounts of complex, unstructured data.
- Deep neural networks enable such devices as voice-controlled virtual assistants; self-driving vehicles, which learn to recognize traffic signs; and computer-vision systems used by doctors to more quickly and accurately assess X-rays and other medical images.
Generative AI: Generative AI is an AI system capable of generating text, images, and other content in response to prompts. Generative AI systems are often built as large and complex neural networks.
- Large language models (LLMs) are the processing engines of text-based generative AI tools. LLMs are trained on immense volumes of text data, in the terabytes range, to assemble words and sentences that solve for the sought-after response with the most probable result. From a user’s prompts (input) the LLM can suggest a solution (output). ChatGPT is an example of an LLM in the form of a chatbot.
How is AI being used to improve medical care and biomedical research?
• Radiology: The ability of AI to interpret imaging results may aid in detecting a minute change in an image that a clinician might accidentally miss.
• Telehealth: Wearable devices allow for continuous monitoring of patients. AI that is integrated into wearable devices can detect physiological changes that could give early warning of a medical event, such as an asthma attack.
• Clinical care: A large focus of AI in the healthcare sector is in clinical decision support systems, which use health observations and the patient’s health history to assist with treatment decisions.
How are AI datasets created?
Huge datasets are needed for AI applications to be developed. For example, to develop an algorithm for medical imaging, the dataset must contain medical images that are curated, de-identified, assessed for quality, annotated, and labelled by clinical experts. This significant effort is necessary to create high quality data sets that can be used to “teach” AI tools. As with any emerging technology there can be risks associated with its use. In creating AI tools, researchers must assure they are both fair and perform equally across groups and populations.
The NIBIB-supported Medical Imaging and Data Resource Center (MIDRC) is an example of high-quality imaging and clinical data that is available to researchers to develop AI tools. Imagine a massive library filled with X-rays, CT scans, and MRIs from patients all over the United States. AI algorithms being developed churn through large volumes of MIDRC data to become proficient and better at analyzing and making predictions. The AI algorithms that are being developed could help doctors make earlier diagnoses, more effective treatment plans, and more precise patient outcomes predictions. Read more about MIDRC at https://www.midrc.org/.
How are NIBIB-funded researchers leading the way in the development and application of AI in biomedicine?
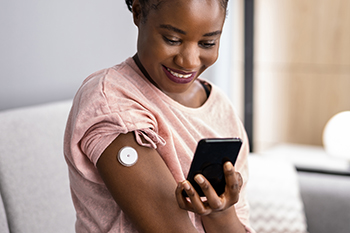
Prediction of blood glucose levels using wearable sensors
NIBIB-funded researchers are using data from wearable sensors to build machine learning models for people to better manage their blood glucose levels. Portable sensing technologies provide continuous heart rate, electrical characteristics of the skin, temperature, and body movement measurements. The data can then be used to train an AI network to predict changes in blood glucose levels. Anticipating and preventing blood glucose control problems can enhance patient safety and reduce costly complications.
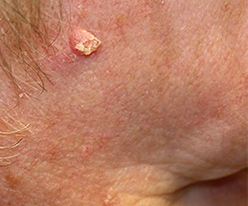
Fighting Skin Cancer with Smarter Treatment through AI risk stratification
Cutaneous squamous cell carcinoma (cSCC) is a common type of skin cancer. Doctors who treat cSCC need a better way to identify patients who would benefit from stronger treatment. An NIBIB supported project uses deep learning to support such decisions. Using a huge collection of images from past skin cancer cases and AI models trained to find patterns in those images, the model can predict how serious a case is and what the optimal treatment would be. The deep learning AI first looks for image features that doctors already know are important. Then, the AI predicts how the cancer will progress. AI has the potential to discover clues about cSCC that doctors have not previously observed.
Illuminating the black box: Explaining Medical AI
Artificial intelligence is revolutionizing healthcare. But many AI models, like proverbial black boxes, do not reveal how the model derives its results. Doctors, researchers, and patients would hesitate to trust AI generated predictions if they could not delve into its reasoning. Therefore, researchers used a technique to match concepts with corresponding clinical images. The AI model links concepts used to describe image characteristics and respective images. The output overcomes bottlenecks of data labeling encountered when training the AI model and allows for human supervision and inspection of how AI reached its conclusions. The approach is part of an area of research called eXplainable AI, or XAI. By making AI models more explainable, XAI is generating trust in how AI is deployed for medical applications, such as in differentiating skin lesions that are benign from those that are cancerous.