Collaborators
Project Brief
Emotions play an important role in decision making. Emotions also influence our autonomic nervous system presumably through the amygdala and other regions of the brain. It has been hypothesized that feedback from autonomic nervous activity enhances learning and decision making. Well-learnt associations are used to predict or anticipate outcomes of related events, thereby increasing the chances of making a correct decision and ultimately increasing the deciding organism’s chances of survival. Animal research in decision making must account for emotional responses without the benefit of using language to probe the subject's experiences. Autonomic measures provide an indirect readout of emotional state, providing researchers insight into the animal's experiences (anticipation and receipt of rewards) during an experiment.
According to neuroeconomic theory, the goal of the nervous system, in evolutionary terms, is to maximize the inclusive fitness of the organism. Recent studies in humans investigated the interplay among valuation, decision making and brain activity. However, animal studies that could provide more detailed studies of the brain are rare, hampered by the difficulty in making “clean” recordings and the difficulty in training cognitive valuation tasks.
Our NIMH collaborators had three primary aims: 1) devise a set of computational tools that automatically mitigate the effects of noise in autonomic recordings of ECG and pupil size, 2) develop tools to demonstrate that a rhesus monkey could learn arbitrarily-assigned values of visual symbols in a decision-making game, and 3) apply these tools to analyze the monkey autonomic nervous activity before and during acquisition of various rewards from the game. While NIMH had the resources to conduct the experiments (including the ECG and pupil size recordings), the nature of the experiment resulted in low quality signals with high noise levels.
SPIS staff developed signal processing algorithms to improve the signal quality of the pupil size and ECG data before analysis by our NIMH collaborators. In addition to reducing the noise to acceptable levels, we performed post-processing tasks such as pupil size data validation (e.g. eliminating trials in which a blink is detected) and heart rate variability analysis.
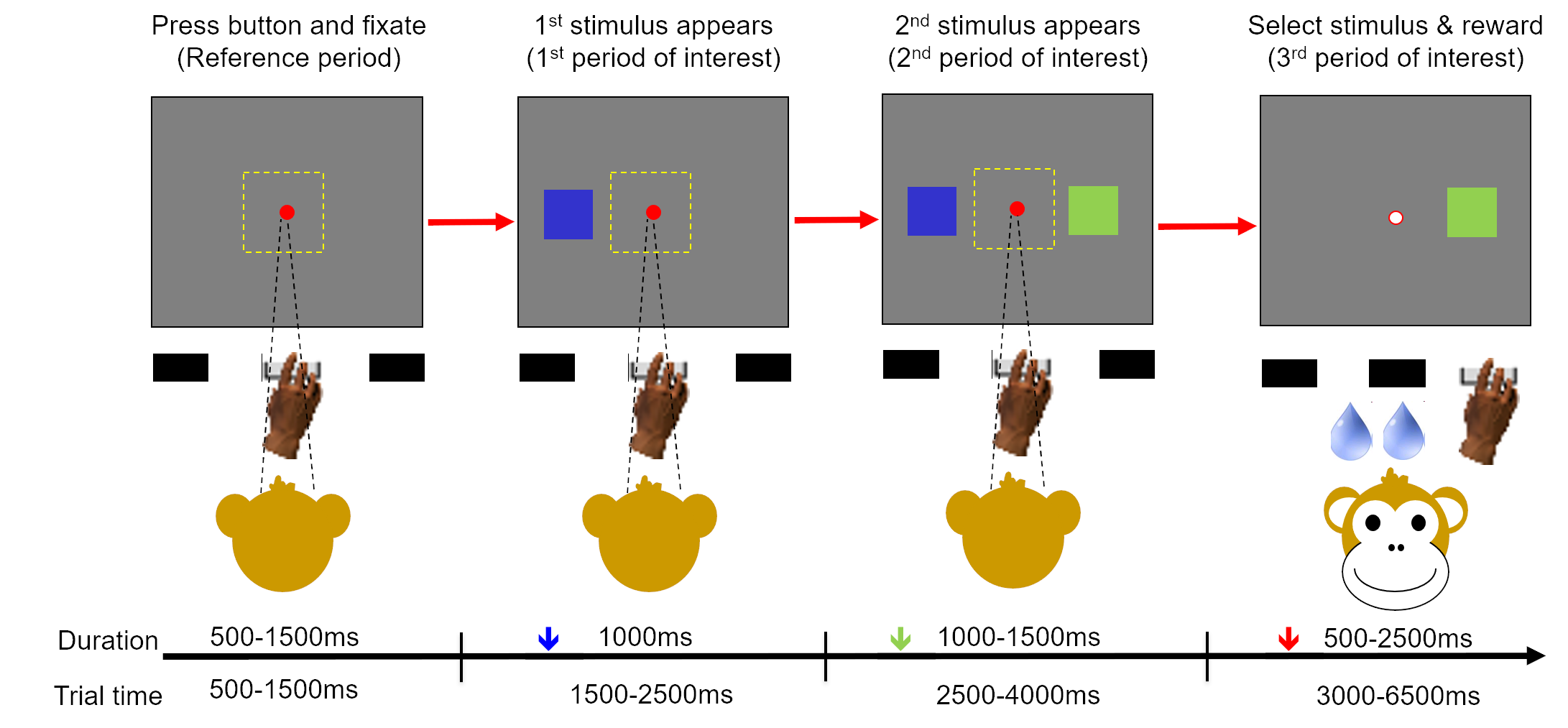
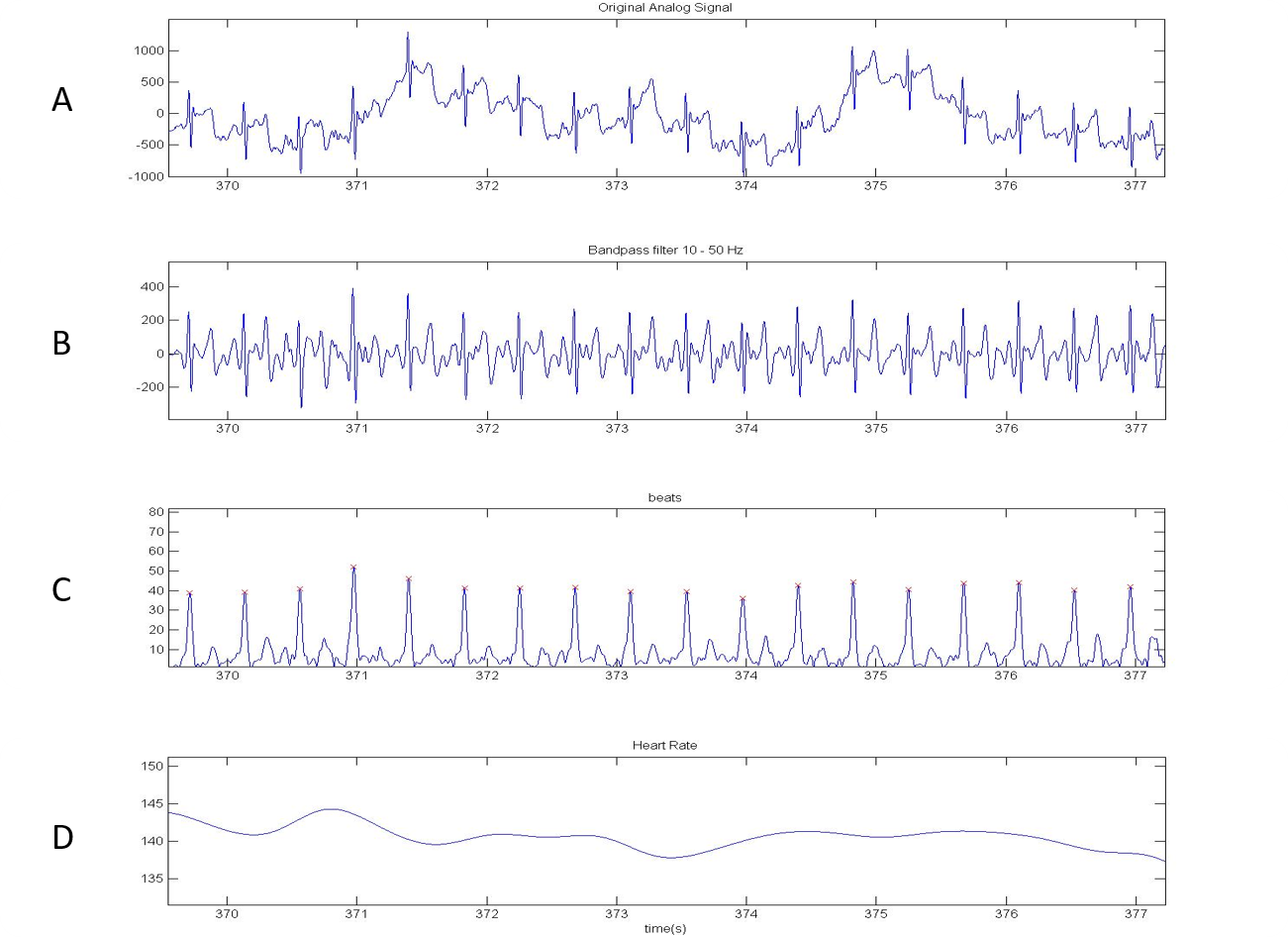
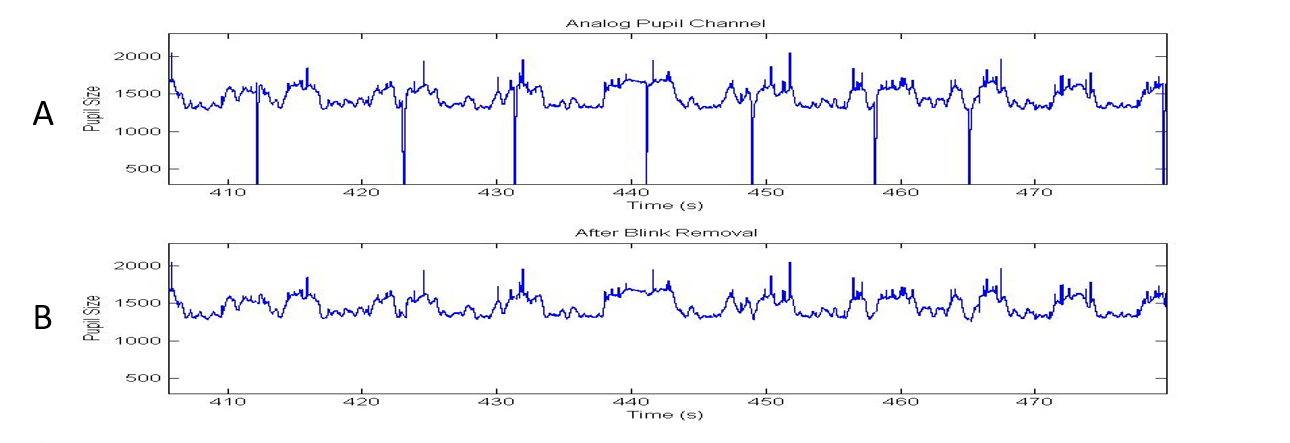