Approach can guide better drug design for cancer, infectious diseases
To counter drug resistance, scientists engineer new drugs to “fit” new mutations and thus kill the cancer cell or pathogen. Now, an NIBIB-funded team of Penn State engineers has a new approach for predicting which mutation has expanded the most in a population and should be targeted to design the most effective new drug.
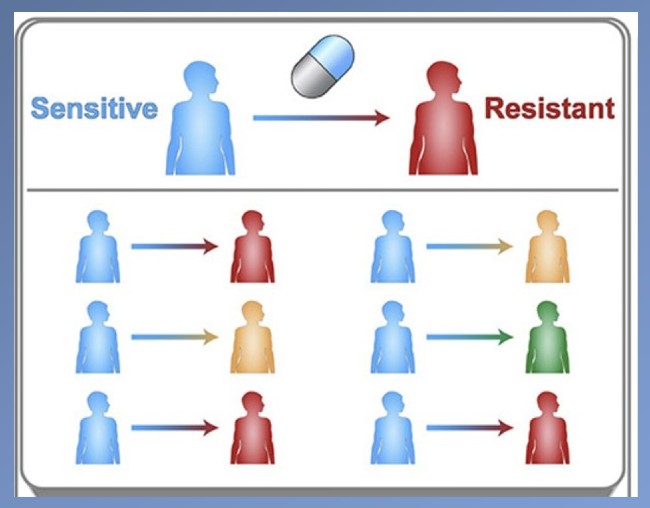
The need to design new drugs that overcome resistance is crucial to fight a mutated virus in a population of people or a mutated protein that causes drug resistance in a population of tumor cells.
“Traditionally, drug developers target the most resistant mutation — the one that completely negates a drug’s effect—assuming it will be the most prevalent,” explained Justin Pritchard, Ph.D., assistant professor of biomedical engineering and holder of the Dorothy Foehr Huck and J. Lloyd Huck Early Career Entrepreneurial Professorship.
However, Pritchard and his team’s computer models have revealed that mutations that have a weaker resistance to the drug can also expand to be the most prevalent in a population of cells. In that case, the mutant that is more prevalent -- even if less resistant -- would need to be targeted to have the greatest effect at treating the disease.
The group tested their approach on mutating cancer cells. In a study of mutations that arose in leukemia patients before and after drug treatment, they found that the mutation that most strongly blocked the effect of the original leukemia drug was not the most common. Instead, a less resistant mutant had expanded to become the most prevalent in the tumor cells. In that case, a new drug targeting the more moderately resistant mutant would be the most effective at targeting the most leukemia cells.
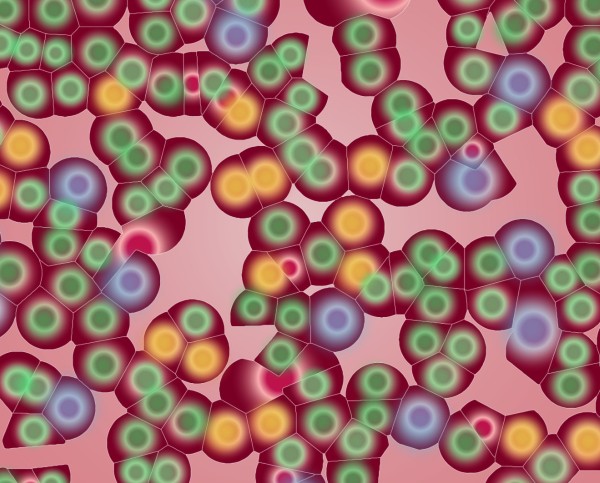
“Similar proteins can be made using slightly different genetic codes,” explained Pritchard. “Our model found that the slight differences caused certain versions of the resistance proteins to be made more efficiently by the cell’s machinery. In the case of leukemia, that type of bias caused the less resistant protein to be the most widely spread in the leukemia cell population.” And thus, though it has a weaker effect, its ubiquity in the population made it more dangerous than the most resistant but less prevalent mutant.
“The leukemia result demonstrated that mutation bias will drive prevalence when drug resistance does not,” said Pritchard, “and verifies that this modeling approach could be extremely useful for designing a drug that would have the best effect in leukemia patients.” The team also found similar results with breast, prostate and stomach cancers, although the effect was not as striking as in the leukemia analysis.
The researchers are continuing to refine their model with the aim of incorporating this type of information into the process of rational drug design. The approach could significantly improve the ongoing challenge of developing drugs that combat resistant cancers as well as resistant bacterial and viral infections that occur when diseases spread throughout a population.
This work was reported in the March issue of Cell Reports1 and supported by an R21 from the National Institute for Biomedical Imaging and Bioengineering (NIBIB) (R21EB026617).