NIBIB-funded researchers are constructing macroscopic medical images of cells and tissues at speeds 7,000 times faster than current methods allow, using a deep learning approach. Producing high-quality, real-time, macroscopic molecular images could enable medical experts to make better, more informed clinical diagnoses. The new technique, by a team at Rensselaer Polytechnic Institute, may produce better images of living organisms in real-time.
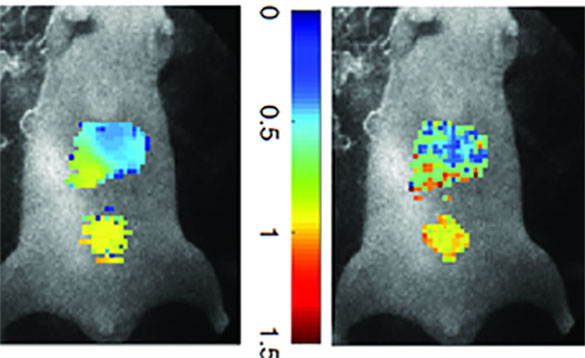
Molecular imaging allows medical experts to observe a detailed picture of what is happening inside a person’s cells and tissues by exploiting various traits of molecules associated with a disease, also known as biomarkers. In optical imaging, scientists attach fluorescent tags to the biomarkers.
They can simultaneously monitor multiple biomarkers by varying the fluorescent colors bound to each one, so they are easy to differentiate from one another. With this technique, they can measure the length of time a molecule stays glowing or in an excited state, defined as ‘lifetime’. Measuring the lifetime of a molecule can be useful for monitoring drug delivery or the environment immediately surrounding the molecule (microenvironment).
The length of time it takes for data-processing “is one of the main barriers in measuring the lifetime of multiple fluorescent biomarkers, especially for clinical applications,” said Behrouz Shabestari, Ph.D., director of the NIBIB program in Artificial Intelligence, Machine Learning, and Deep Learning. “Developments in artificial intelligence in biomedical imaging, especially deep learning, are beginning to significantly improve image quality and speed up processing times.”
Deep learning is a subset of machine learning that is an assembly of artificial neural networks, often compared to the human brain. A network consists of many nodes, loosely akin to neurons in the brain. An assigned weight amplifies or reduces input from a node, depending on the assigned task.
Pingkun Yan, Ph.D., co-director of the Biomedical Imaging Center at Rensselaer explained, “Nodes are the sites where computation takes place – they are like filters in convolutional neural networks.” He described convolutional neural networks as many layers of nodes that are stacked together, and data is fed through the network one layer at a time; but, like neurons in your brain, not every node is activated for each task. The network must be trained with a large amount of data to be successful at processing information.
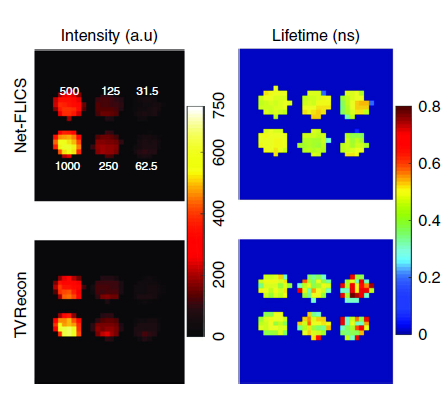
Deep learning has been applied to biomedical imaging to improve the quality of an existing image for improved clinical diagnosis. “But what our team is working towards is unique; we are using deep learning to execute the physics behind the original construction of the image,” explained Xavier Intes, Ph.D., co-director of the Biomedical Imaging Center at Rensselaer and the corresponding author of the paper published in the Nature journal Light: Science and Applications.
Building upon a previous approach developed by the same team, the new technique called Net-FLICS (fluorescence lifetime imaging with compressed sensing), uses deep learning to construct an image from a small number of point measurements.
Intes’ and Yan’s team created an entirely new deep learning network to build molecular images. Often, a network that is exceptional at identifying images in a google search can be slightly adapted for similar applications like facial recognition; this process is called transfer learning. “There wasn’t an existing network for our specific application, so we had to take pieces of other networks to build what we needed,” said Yan.
The researchers trained the network using simulations and computer models to generate a large amount of data. Using models gave the team an enormous advantage – they had access to an infinite amount of data, which is a common obstacle in training a network.
However, there are challenges to working with a new network. “It’s hard to comprehend what occurs inside a brand-new network like ours – it’s kind of like a black box. As we continue to learn more, it will become clearer why the network makes certain decisions, and we can improve it,” explained Intes.
The significant advantages of Net-FLICS allow images to be acquired in almost real-time, opening new doors to researchers and clinicians due to the improved speed and quality of images. Future applications of the Net-FLICS technique will help identify which individual cells show uptake of drug treatment, and which ones do not. Real-time molecular imaging could also aid surgeons while performing complicated procedures like removing a tumor or working around delicate nerves.
This work was supported by the National Institutes of Health Grants R01 EB19443 and R01 CA207725.
Yao R, Ochoa M, Yan P, Intes X. Net-FLICS: fast quantitative wide-field fluorescence lifetime imaging with compressed sensing - a deep learning approach. Light Sci Appl. 2019; 8:26. Published 2019. doi:10.1038/s41377-019-0138-x