This ability could lead to unintended biases in AI models
Artificial intelligence, or AI, is used in a wide variety of health care settings, from analyzing medical images to assisting with surgical procedures. While AI can sometimes outperform trained clinicians, these superhuman abilities are not always fully understood.
In a recent study, published in Lancet Digital Health, NIH-funded researchers found that AI models could accurately predict self-reported race in several different types of radiographic images—a task not possible for human experts. These findings suggest that race information could be unknowingly incorporated into image analysis models, which could potentially exacerbate racial disparities in the medical setting.
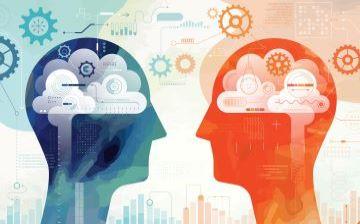
Credit: iStock
“AI has immense potential to revolutionize the diagnosis, treatment, and monitoring of numerous diseases and conditions and could dramatically shape the way that we approach health care,” said first study author and NIBIB Data and Technology Advancement (DATA) National Service Scholar Judy Gichoya, M.D. “However, for AI to truly benefit all patients, we need a better understanding of how these algorithms make their decisions to prevent unintended biases.”
The concept of bias in AI algorithms is not new. Research studies have shown that the performance of AI can be affected by demographic characteristics, including race. There are several potential factors that could lead to bias in AI algorithms, such as using datasets that are not representative of a patient population (e.g., using datasets where most patients are white). Further, confounders—traits or phenotypes that are disproportionately present in subgroup populations (such as racial differences in breast or bone density)—can also introduce bias. The current study highlights another potential factor that could introduce unintended biases into AI algorithms.
For their study, Gichoya and colleagues first wanted to determine if they could develop AI models that could detect race solely from chest x-rays. They used three large datasets that spanned a diverse patient population and found that their models could predict race with high accuracy—a striking finding, as human experts are unable to make such predictions by looking at x-rays. The researchers also found that the AI could determine self-reported race even when the images were highly degraded or cropped to one ninth of the original size, or when the resolution was modified to such an extent that the images were barely recognized as x-rays. The research team subsequently used other non-chest x-ray datasets including mammograms, cervical spine radiographs, and chest computed tomography (CT) scans, and found that the AI could still determine self-reported race, regardless of the type of scan or anatomic location.
“Our results suggest that there are ‘hidden signals’ in medical images that lead the AI to predict race,” said Gichoya. “We need to accelerate our understanding of why these algorithms have this ability, so that the downstream applications of AI—such as building image-based algorithms to make predictions about health—are not potentially harmful for minority and underserved patient populations.”
The researchers attempted to understand how the AI was able to make these predictions. They looked at a variety of different confounders that could potentially affect features in radiographic images, such as body mass index (BMI), breast density, bone density, or disease distribution. They could not identify any specific factor that could explain the ability of AI to accurately predict self-reported race. In short, while AI can be trained to predict race from medical images, the information that the models use to make these predictions has yet to be uncovered.
“There has been a line of thought that if developers ‘hide’ demographic factors—like race, gender, or socioeconomic status—from the AI model, that the resulting algorithm will not be able to discriminate based on such features and will therefore be ‘fair.’ This work highlights that this simplistic view is not a viable option for assuring equity in AI and machine learning,” said NIBIB DATA Scholar Rui Sá, Ph.D. “We need to recognize the potential limitations of AI and adapt our methodologies to ensure that AI is fair for all.”
The study reported here is part of MIDRC (The Medical Imaging Data Resource Center) and was made possible by NIBIB under contracts 75N92020C00008 and 75N92020C00021. This study was also supported with additional funding from NIBIB (grant EB017205), funding from the National Library of Medicine (NLM; grant LM012966), and funding from the National Science Foundation (NSF).
Study reference: Gichoya, Judy Wawira et al. AI recognition of patient race in medical imaging: a modelling study. The Lancet Digital Health, 2022; 4(6): 406-414. doi: 10.1016/S2589-7500(22)00063-2