Through device engineering and biological engineering, this division supports the development of broadly applicable biomedical technologies (without preference for any disease or application) that directly interface with, monitor, and regulate biological processes and functions of human physiology to enable new paradigms of human health. This division also supports research to advance the design and deployment of future technology-driven interventions.
Program Areas
Biological and Materials Engineering Programs
Collaborations
An important aspect of the Institute’s mission is encouraging collaborations among the institutes and centers at NIH, other federal agencies, and the private sector.
- Interagency Modeling and Analysis Group (IMAG) – IMAG brings together program officers across multiple federal agencies to communicate, disseminate, and plan collaborative activities and joint initiatives related to computational modeling and analysis of biomedical, biological, and behavioral systems. IMAG coordinates the Multi-scale Modeling (MSM) Consortium, see IMAG wiki.
- Synthetic Biology Consortium (SBC) - The SBC is a trans-NIH effort to foster collaborations among researchers and share synthetic biology technologies to address unmet needs in biology and medicine.
- Biomaterials Network - The Biomaterials Network consists of a set of connected resources that enable novel and refined technologies to progress through discovery and development to commercialization and clinical use.
Division Staff
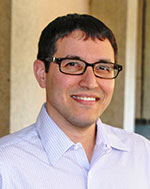
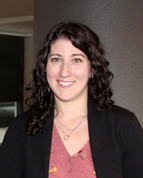
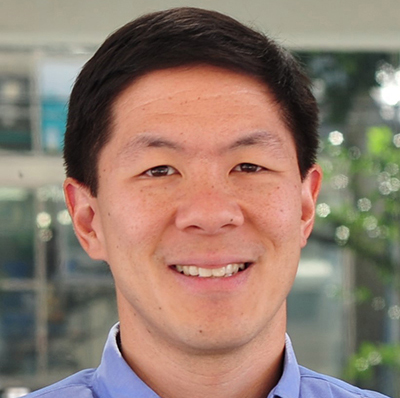
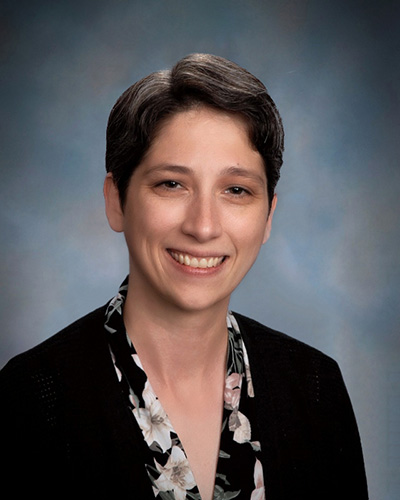
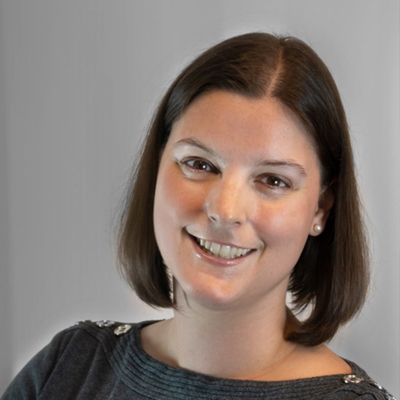
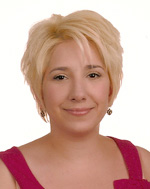
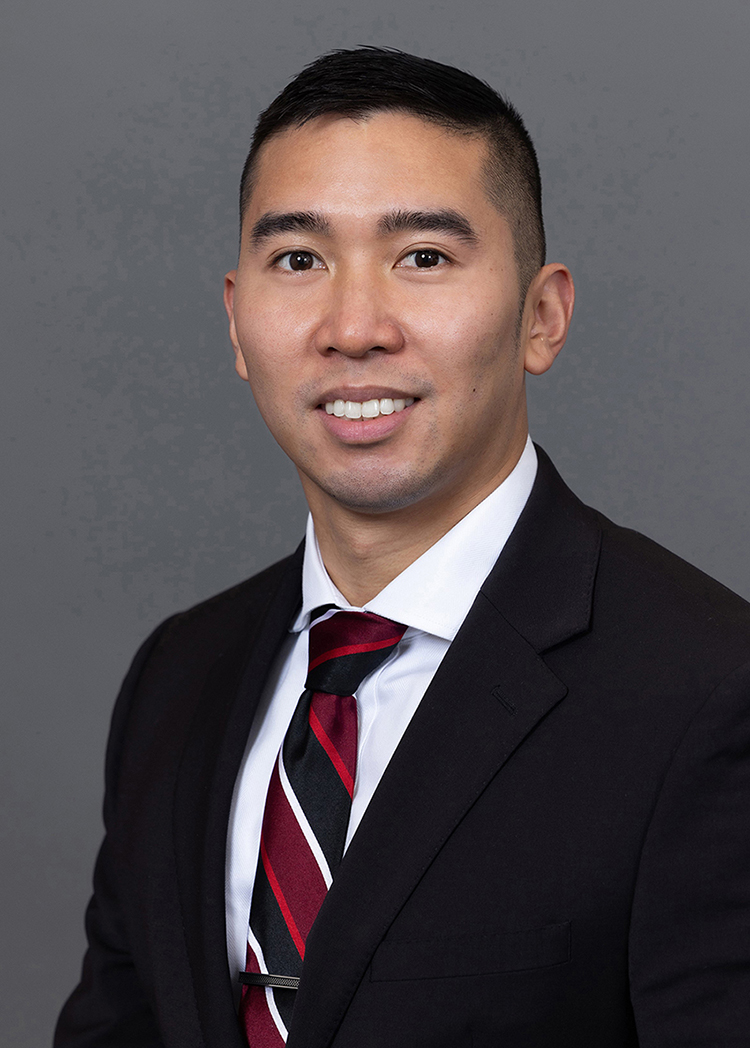
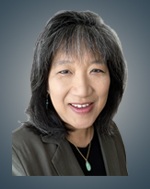
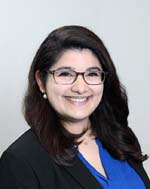