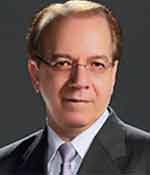
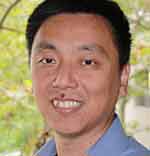
Emphasis
The emphasis is on using image data to achieve better health outcomes and smarter health care. Examples of technology development areas in this program include but are not limited to models, algorithms, software, methodologies, and other tools that will: facilitate medical imaging research; support clinical detection, diagnosis and therapy; and improve patient healthcare.
Program priorities and areas of interest:
- Image segmentation, image registration, atlas generation, image fusion, morphometry measurement, and the determination of function and structure from medical images
- Diagnostic-performance evaluation, computer-aided diagnosis, statistical models for evaluation of observer performance, and assessment of observer variability
- Quantitative imaging and image-based biomarkers
- Image-driven computer-aided diagnosis and decision support systems
- Virtual reality technologies
- Dose estimation and reduction software
Additional support
This program also supports:
- Early-stage validation of tools for image processing, visual perception and display
- Tools to assess image quality and observer performance
- Tools and software that enable large-scale, longitudinal and/or multi-site imaging studies and clinical trials
- Medical imaging mobile apps for early detection
Related News
NIH Blueprint MedTech program has issued nine awards in its first competition cycle. The program seeks to accelerate transformative medical devices to treat disorders of the nervous system.
NIH will advance the development of home-based and point-of-care health technologies with awards to six technology research and development centers around the country. The centers comprise the Point of Care Technology Research Network (POCTRN) and will parlay the momentum of the original network established in 2007 by the NIBIB.
New recommendations from the NIH Rapid Acceleration of Diagnostics (RADx®) Tech Program provide a blueprint for the design and manufacture of more accessible diagnostic tests.
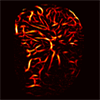
Researchers have shown that an automated cancer diagnostic method, which pairs cutting-edge ultrasound techniques with artificial intelligence, can accurately diagnose thyroid cancer, of which there are more than 40,000 new cases every year.
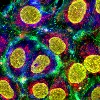
NIBIB researchers and their collaborators introduce several novel image restoration strategies that create sharp images with significantly reduced processing time and computing power.